My dissertation “Experimental explorations of a discrimination learning approach to language
processing” ()
investigates the potential of discrimination learning for understanding the way in which humans process language.
Below, I summarize the main findings of my dissertation in a way that, hopefully, is accessible to a broad audience.
Every day, we use language to communicate about the world around us in a seemingly effortless manner. Without any significant problems, we understand others and others understand us as we convey information about countless objects and events in this world. Rarely, if ever, do we ask ourselves the question “How is this possible?”.
Psycholinguistics is a field of research that tries to answer this and many more questions about the human language processing system. Typically, data are collected through experiments in a lab, in which participants are asked to complete a linguistic task while their behavior is tracked in one form or another. Subsequently, the data from these experiments are analyzed in an attempt to gain new insights into linguistic processing.
Oftentimes, psycholinguistic researchers investigate the effects of lexical distributional variables on behavioral measures of language processing. Lexical distributional variables are measures that describe the distributional properties of a linguistic stimulus, such as the frequency of occurrence of a word or the number of words that are similar in form to a word (e.g., “life” is similar to “wife”). The effects of lexical distributional variables inform us about which properties of linguistic stimuli influence language processing. They provide no information, however, about why these properties - and not others - are important.
While lexical distributional variables provide different higher-level windows on the language processing system, my dissertation is an attempt to describe the language processing system itself. Unlike analyses and linguistic models that are based on lexical distributional variables, it takes into account the role of learning. The point of departure for the analyses of linguistic data sets presented in my dissertation is a simple general-purpose probabilistic learning algorithm (cf. Chater et al., 2006; Hsu et al., 2010, see also Baayen et al., 2011): the Rescorla-Wagner equations (Rescorla & Wagner, 1972). As a mathematical formalization of discrimination learning, the Rescorla-Wagner equations describe how people learn to respond differently to different stimuli, be they linguistic or non-linguistic in nature.
The Rescorla-Wagner equations describe a two-layer network model, in which both input units and outcomes are symbols. In the work presented in my dissertation, these symbols are linguistic units, such as letters, phonemes or words. As such, the symbolic approach used in my dissertation stands in contrast to sub-symbolic approaches, in which linguistic units are represented as activation patterns over non-symbolic units (which, at a lower level of granularity, are again symbolic). Symbolic models are an oversimplification of a more complex neurobiological reality (as are many implementations of sub-symbolic models), but provide highly competitive performance and an increased interpretability as compared to sub-symbolic models of language processing.
More precisely, the foundation of the work presented in my dissertation was laid down in Baayen et al. (2011), who describe an implementation of - the equilibrium equations for Danks (2003) - the Rescorla-Wagner equations in a model for silent reading. Given that the associations between input units and outcomes were estimated independently for each outcome - an assumption similar to the independence assumption in a statistical classification technique referred to as Naive Bayesian Classifiers - Baayen et al. (2011) refer to their model as the Naive Discriminative Reader (NDR). The NDR model accounted for a wide range of effects documented in the experimental reading literature.
The NDR model, however, was limited to silent reading. To truly gauge the potential of a computational approach to language processing, it is pivotal to investigate its performance across a variety of experimental tasks and the behavioral measures of language processing obtained through these tasks. My dissertation provides a more extensive evaluation of the possibilities offered by a discrimination learning approach to language processing, by looking at the explanatory power of discrimination learning networks in three different experimental tasks and for three different dependent variables.
First, my thesis presents an extension of the NDR model for silent reading to reading aloud. The basic architecture of the resulting Naive Discriminative Reading Aloud (NDRa) model is shown in Figure 1. The model consists of two discrimination learning networks. The first network maps orthographic features onto lexico-semantic representations, similar to the discrimination learning network for silent reading described in Baayen et al. (2011). The second network maps lexico-semantic representations onto phonological features.
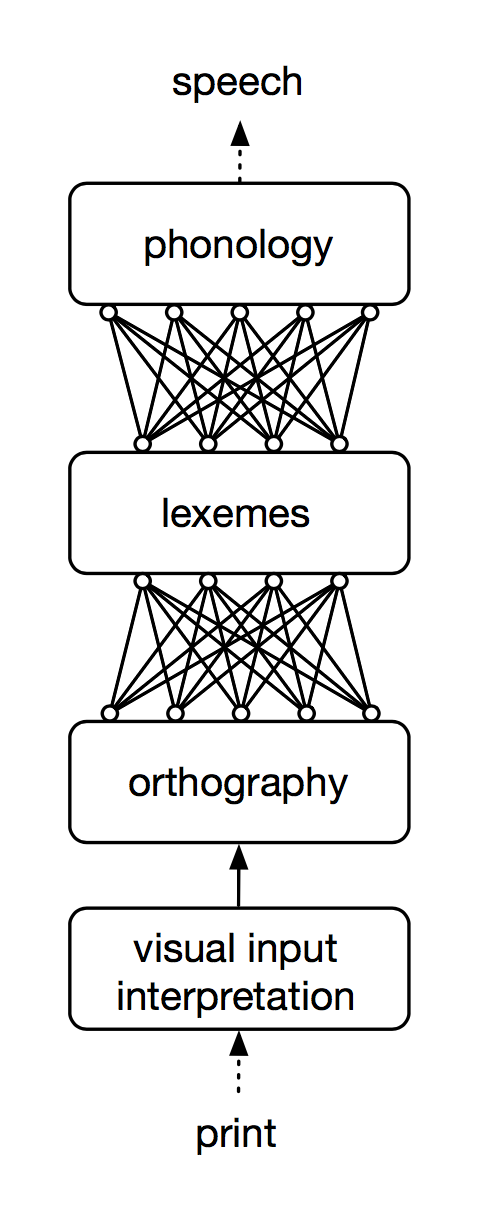
Figure 1: basic architecture of the NDRa model
Existing models of reading aloud typically consist of two routes: a lexical route in which the orthography-to-phonology mapping is mediated by lexico-semantic representations and a sub-lexical route, in which orthographic units are mapped directly onto phonological units. By contrast, a single lexical architecture is responsible for both word and non-word naming in the NDRa model. In word reading, the orthographic presentation of the target word (e.g., “life”) activates the lexico-semantic representation of the target word, as well as the lexico-semantic representations of orthographically similar words (e.g., “wife”, “knife”). These lexico-semantic representations then activate phonological units, which allow for the pronunciations of the target word. For a non-word (e.g., “kife”), no lexico-semantic representation exists. The activation of phonological units, therefore, is driven exclusively by the activation of orthographic neighbors of the non-word (e.g., “life”, “wife”, “knife”).
An extensive evaluation of the NDRa model demonstrates that the single-route architecture of the NDRa model is capable of capturing a wide range of effects documented in the reading aloud literature, both for words and for non-words, including linear and non-linear effects of neighborhood density measures and the consistency of the orthography to phonology mapping, as well as a hitherto unobserved effects of non-word frequency. Despite its much more parsimonious model architecture, the overall performance of the NDRa model is highly similar to that of a state-of-the-art dual-route model of reading aloud (see Perry et al., 2007). Furthermore, the addition of a sub-lexical route architecture does not further improve the performance of the NDRa model. When using a discrimination learning approach, therefore, a single lexical route is sufficient to provide a highly competitive account of language processing in the reading aloud task.
The second test-case for a discrimination learning approach presented in my dissertation is an investigation of the eye fixation patterns during noun-noun compound reading in a new large-scale corpus of eye movements during natural discourse reading, the Edmonton-Tübingen eye-tracking corpus (ET corpus). An analysis using distributional lexical variables indicates that the fixation patterns on compounds in the ET corpus do not fit straightforwardly with existing sub-lexical (constituent access precedes full-form access), supra-lexical (full-form access precedes constituent access) or dual-route (a holistic and a decompositional route are pursued in parallel) models of compound reading. An analysis using predictors derived from two naive discrimination learning (henceforth NDL) networks sheds further light on the processes that drive compound reading and suggests that compound reading is perhaps better thought of as an attempt to activate the lexico-semantic information associated with a compound given all information available to the reader (cf. maximization of opportunities, Libben, 2006).
Over 60% of the time, a single fixation on a compound suffices. During single fixations, readers fixate far enough into a compound to make all orthographic features of the compound available. These orthographic features then activate all lexico-semantic information associated with a compound: first-and-only fixation durations are co-determined by an integrative measure of the bottom-up support for the lexico-semantic representations of not only the compound as a whole, but also the modifier and the head.
Nearly 40% of the time, readers need a second fixation to successfully process a compound. An important cause of additional fixations is a suboptimal fixation position during the first fixation. As a result, not all orthographic features of a compound are available to the reader during first-of-many fixations. Accordingly, the NDL measure that proved most predictive for first-and-only fixation duration is the activation of the lexico-semantic representation of the modifier given the first orthographic trigram of a compound only.
In the analysis using lexical distributional variables, both first-and-only and second fixation durations were influenced by the frequency of the compound. On the basis of a lexical predictor analysis one might therefore be tempted to conclude that the processes underlying single fixations and second fixations are similar. The NDL analysis of the compound reading data, however, demonstrates that the frequency effects for first-and-only fixations and second fixations are qualitatively different. Whereas first-and-only fixation durations are characterized by the bottom-up support for the lexico-semantic information associated with a compound, second fixation durations are influenced by the out-of-context, a priori probability of a compound. In other words: during second fixations readers resort to a top-down “best guess” strategy.
Overall, the explanatory power of lexical distributional variables and that of NDL measures was highly similar. As for the reaction times in the reading aloud task, therefore, the NDL framework offers a highly competitive perspective on eye fixation patterns during compound reading. As demonstrated above, however, the NDL measures provide more detailed and more differentiated insights into the processes that underlie these fixation patterns.
Third, the perhaps most stringent test of the potential of discrimination learning in my dissertation is an exploration of the explanatory power of NDL measures for the electroencephalographic correlates of language processing in a primed picture naming task, as gauged through the ERP signal following picture onset. In this primed picture naming task, participants were presented with preposition plus definite article primes (e.g., “on the”) and target pictures of concrete nouns (e.g., “STRAWBERRY”). The data for this primed picture naming experiment show an effect for preposition frequency, theta range oscillations for word frequency and constructional prototypicality, and a prolonged near-linear effect for phrase frequency.
An NDL analysis of the ERP data shows an effect of the bottom-up support for preposition that qualitatively and topographically resembles the effect of preposition frequency. Furthermore, the effect for the bottom-up support for the target word shows some similarities to the effect of word frequency. Most similar to the effect of word frequency, however, is the effect of top-down information about the a priori probability of the target word. Again, therefore, the NDL analysis provides more detailed insight into the nature of a frequency effect.
The quantitive performance of the NDL measures in explaining the ERP signal after picture onset is at least as good as the performance of lexical distributional variables. In a regression analysis NDL measures and lexical predictors explain a very similar amount of the variance in the ERP signal. According to an analysis with a tree-based machine learning technique, however, NDL measures significantly outperform lexical distributional variables.
In conclusion, my dissertation assesses the potential of discrimination learning as a perspective on the adult language processing system. Across three experimental tasks and three behavioral measures of language processing, discrimination learning shows highly competitive performance as compared to existing analysis techniques and models of language processing, and allows for new or more refined insights into the systemic properties that drive language processing through a general-purpose learning mechanism that is remarkably simple and transparent.
Baayen, R. H., Milin, P., Filipović-Đurđević, D., Hendrix, P. & Marelli, M. (2011). An amorphous model for morphological processing in visual comprehension based on naïve discrimination learning. Psychological Review, 118, 438-482.
Chater, N., Tenenbaum, J. B. & Yuille, A. (2006). Probabilistic models of cognition: Conceptual foundations. Trends in Cognitive Science,10(7), 287-291.
Hsu, A. S., Chater, N. & Vitányi, P. (2011). The probabilistic analysis of language acquisition: Theoretical, computational, and experimental analysis. Cognition, 120, 380-390.
Rescorla, R. & Wagner, A. (1972). A theory of Pavlovian conditioning: Variations in the e ectiveness of reinforcement and nonreinforcement. In A. H. Black & W. F. Prokasy (Eds.), Classical conditioning II (pp. 64-99). New York: Appleton-Century-Crofts.
Perry, C., Ziegler, J. & Zorzi, M. (2007). Nested incremental modeling in the development of computational theories: the CDP+ model of reading aloud. Psychological Review, 114(2), 273-315.
Libben, G. (2006). Why study compound processing? In G. Libben & G. Jarema (Eds.), The representation and processing of compound words (pp. 1-23). Oxford: Oxford University Press.
Danks, D. (2003). Equilibria of the Rescorla-Wagner model. Journal of Mathematical Psychology, 47(2), 109–121.
Every day, we use language to communicate about the world around us in a seemingly effortless manner. Without any significant problems, we understand others and others understand us as we convey information about countless objects and events in this world. Rarely, if ever, do we ask ourselves the question “How is this possible?”.
Psycholinguistics is a field of research that tries to answer this and many more questions about the human language processing system. Typically, data are collected through experiments in a lab, in which participants are asked to complete a linguistic task while their behavior is tracked in one form or another. Subsequently, the data from these experiments are analyzed in an attempt to gain new insights into linguistic processing.
Oftentimes, psycholinguistic researchers investigate the effects of lexical distributional variables on behavioral measures of language processing. Lexical distributional variables are measures that describe the distributional properties of a linguistic stimulus, such as the frequency of occurrence of a word or the number of words that are similar in form to a word (e.g., “life” is similar to “wife”). The effects of lexical distributional variables inform us about which properties of linguistic stimuli influence language processing. They provide no information, however, about why these properties - and not others - are important.
While lexical distributional variables provide different higher-level windows on the language processing system, my dissertation is an attempt to describe the language processing system itself. Unlike analyses and linguistic models that are based on lexical distributional variables, it takes into account the role of learning. The point of departure for the analyses of linguistic data sets presented in my dissertation is a simple general-purpose probabilistic learning algorithm (cf. Chater et al., 2006; Hsu et al., 2010, see also Baayen et al., 2011): the Rescorla-Wagner equations (Rescorla & Wagner, 1972). As a mathematical formalization of discrimination learning, the Rescorla-Wagner equations describe how people learn to respond differently to different stimuli, be they linguistic or non-linguistic in nature.
The Rescorla-Wagner equations describe a two-layer network model, in which both input units and outcomes are symbols. In the work presented in my dissertation, these symbols are linguistic units, such as letters, phonemes or words. As such, the symbolic approach used in my dissertation stands in contrast to sub-symbolic approaches, in which linguistic units are represented as activation patterns over non-symbolic units (which, at a lower level of granularity, are again symbolic). Symbolic models are an oversimplification of a more complex neurobiological reality (as are many implementations of sub-symbolic models), but provide highly competitive performance and an increased interpretability as compared to sub-symbolic models of language processing.
More precisely, the foundation of the work presented in my dissertation was laid down in Baayen et al. (2011), who describe an implementation of - the equilibrium equations for Danks (2003) - the Rescorla-Wagner equations in a model for silent reading. Given that the associations between input units and outcomes were estimated independently for each outcome - an assumption similar to the independence assumption in a statistical classification technique referred to as Naive Bayesian Classifiers - Baayen et al. (2011) refer to their model as the Naive Discriminative Reader (NDR). The NDR model accounted for a wide range of effects documented in the experimental reading literature.
The NDR model, however, was limited to silent reading. To truly gauge the potential of a computational approach to language processing, it is pivotal to investigate its performance across a variety of experimental tasks and the behavioral measures of language processing obtained through these tasks. My dissertation provides a more extensive evaluation of the possibilities offered by a discrimination learning approach to language processing, by looking at the explanatory power of discrimination learning networks in three different experimental tasks and for three different dependent variables.
First, my thesis presents an extension of the NDR model for silent reading to reading aloud. The basic architecture of the resulting Naive Discriminative Reading Aloud (NDRa) model is shown in Figure 1. The model consists of two discrimination learning networks. The first network maps orthographic features onto lexico-semantic representations, similar to the discrimination learning network for silent reading described in Baayen et al. (2011). The second network maps lexico-semantic representations onto phonological features.
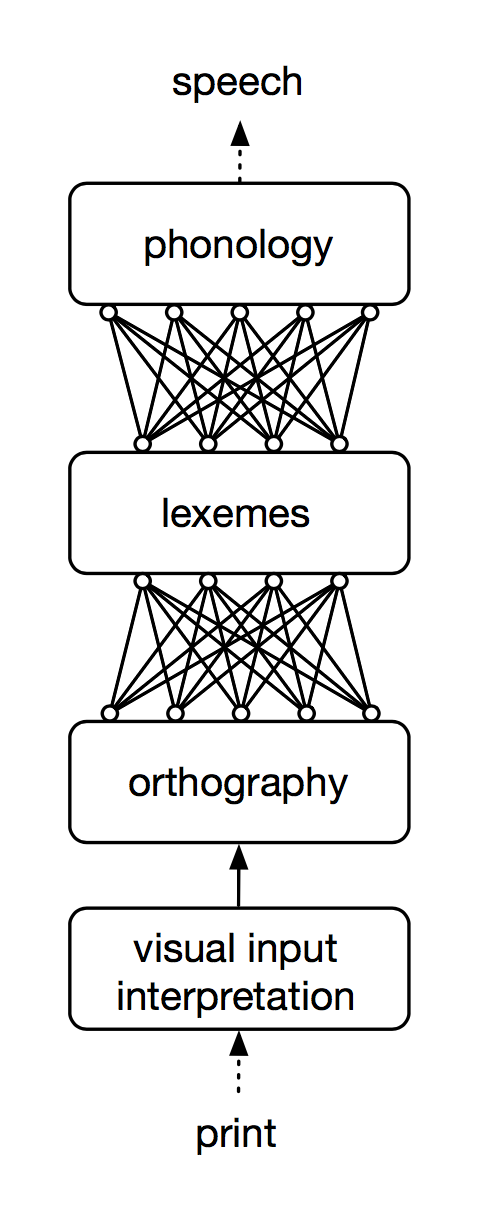
Figure 1: basic architecture of the NDRa model
Existing models of reading aloud typically consist of two routes: a lexical route in which the orthography-to-phonology mapping is mediated by lexico-semantic representations and a sub-lexical route, in which orthographic units are mapped directly onto phonological units. By contrast, a single lexical architecture is responsible for both word and non-word naming in the NDRa model. In word reading, the orthographic presentation of the target word (e.g., “life”) activates the lexico-semantic representation of the target word, as well as the lexico-semantic representations of orthographically similar words (e.g., “wife”, “knife”). These lexico-semantic representations then activate phonological units, which allow for the pronunciations of the target word. For a non-word (e.g., “kife”), no lexico-semantic representation exists. The activation of phonological units, therefore, is driven exclusively by the activation of orthographic neighbors of the non-word (e.g., “life”, “wife”, “knife”).
An extensive evaluation of the NDRa model demonstrates that the single-route architecture of the NDRa model is capable of capturing a wide range of effects documented in the reading aloud literature, both for words and for non-words, including linear and non-linear effects of neighborhood density measures and the consistency of the orthography to phonology mapping, as well as a hitherto unobserved effects of non-word frequency. Despite its much more parsimonious model architecture, the overall performance of the NDRa model is highly similar to that of a state-of-the-art dual-route model of reading aloud (see Perry et al., 2007). Furthermore, the addition of a sub-lexical route architecture does not further improve the performance of the NDRa model. When using a discrimination learning approach, therefore, a single lexical route is sufficient to provide a highly competitive account of language processing in the reading aloud task.
The second test-case for a discrimination learning approach presented in my dissertation is an investigation of the eye fixation patterns during noun-noun compound reading in a new large-scale corpus of eye movements during natural discourse reading, the Edmonton-Tübingen eye-tracking corpus (ET corpus). An analysis using distributional lexical variables indicates that the fixation patterns on compounds in the ET corpus do not fit straightforwardly with existing sub-lexical (constituent access precedes full-form access), supra-lexical (full-form access precedes constituent access) or dual-route (a holistic and a decompositional route are pursued in parallel) models of compound reading. An analysis using predictors derived from two naive discrimination learning (henceforth NDL) networks sheds further light on the processes that drive compound reading and suggests that compound reading is perhaps better thought of as an attempt to activate the lexico-semantic information associated with a compound given all information available to the reader (cf. maximization of opportunities, Libben, 2006).
Over 60% of the time, a single fixation on a compound suffices. During single fixations, readers fixate far enough into a compound to make all orthographic features of the compound available. These orthographic features then activate all lexico-semantic information associated with a compound: first-and-only fixation durations are co-determined by an integrative measure of the bottom-up support for the lexico-semantic representations of not only the compound as a whole, but also the modifier and the head.
Nearly 40% of the time, readers need a second fixation to successfully process a compound. An important cause of additional fixations is a suboptimal fixation position during the first fixation. As a result, not all orthographic features of a compound are available to the reader during first-of-many fixations. Accordingly, the NDL measure that proved most predictive for first-and-only fixation duration is the activation of the lexico-semantic representation of the modifier given the first orthographic trigram of a compound only.
In the analysis using lexical distributional variables, both first-and-only and second fixation durations were influenced by the frequency of the compound. On the basis of a lexical predictor analysis one might therefore be tempted to conclude that the processes underlying single fixations and second fixations are similar. The NDL analysis of the compound reading data, however, demonstrates that the frequency effects for first-and-only fixations and second fixations are qualitatively different. Whereas first-and-only fixation durations are characterized by the bottom-up support for the lexico-semantic information associated with a compound, second fixation durations are influenced by the out-of-context, a priori probability of a compound. In other words: during second fixations readers resort to a top-down “best guess” strategy.
Overall, the explanatory power of lexical distributional variables and that of NDL measures was highly similar. As for the reaction times in the reading aloud task, therefore, the NDL framework offers a highly competitive perspective on eye fixation patterns during compound reading. As demonstrated above, however, the NDL measures provide more detailed and more differentiated insights into the processes that underlie these fixation patterns.
Third, the perhaps most stringent test of the potential of discrimination learning in my dissertation is an exploration of the explanatory power of NDL measures for the electroencephalographic correlates of language processing in a primed picture naming task, as gauged through the ERP signal following picture onset. In this primed picture naming task, participants were presented with preposition plus definite article primes (e.g., “on the”) and target pictures of concrete nouns (e.g., “STRAWBERRY”). The data for this primed picture naming experiment show an effect for preposition frequency, theta range oscillations for word frequency and constructional prototypicality, and a prolonged near-linear effect for phrase frequency.
An NDL analysis of the ERP data shows an effect of the bottom-up support for preposition that qualitatively and topographically resembles the effect of preposition frequency. Furthermore, the effect for the bottom-up support for the target word shows some similarities to the effect of word frequency. Most similar to the effect of word frequency, however, is the effect of top-down information about the a priori probability of the target word. Again, therefore, the NDL analysis provides more detailed insight into the nature of a frequency effect.
The quantitive performance of the NDL measures in explaining the ERP signal after picture onset is at least as good as the performance of lexical distributional variables. In a regression analysis NDL measures and lexical predictors explain a very similar amount of the variance in the ERP signal. According to an analysis with a tree-based machine learning technique, however, NDL measures significantly outperform lexical distributional variables.
In conclusion, my dissertation assesses the potential of discrimination learning as a perspective on the adult language processing system. Across three experimental tasks and three behavioral measures of language processing, discrimination learning shows highly competitive performance as compared to existing analysis techniques and models of language processing, and allows for new or more refined insights into the systemic properties that drive language processing through a general-purpose learning mechanism that is remarkably simple and transparent.
References
Baayen, R. H., Milin, P., Filipović-Đurđević, D., Hendrix, P. & Marelli, M. (2011). An amorphous model for morphological processing in visual comprehension based on naïve discrimination learning. Psychological Review, 118, 438-482.
Chater, N., Tenenbaum, J. B. & Yuille, A. (2006). Probabilistic models of cognition: Conceptual foundations. Trends in Cognitive Science,10(7), 287-291.
Hsu, A. S., Chater, N. & Vitányi, P. (2011). The probabilistic analysis of language acquisition: Theoretical, computational, and experimental analysis. Cognition, 120, 380-390.
Rescorla, R. & Wagner, A. (1972). A theory of Pavlovian conditioning: Variations in the e ectiveness of reinforcement and nonreinforcement. In A. H. Black & W. F. Prokasy (Eds.), Classical conditioning II (pp. 64-99). New York: Appleton-Century-Crofts.
Perry, C., Ziegler, J. & Zorzi, M. (2007). Nested incremental modeling in the development of computational theories: the CDP+ model of reading aloud. Psychological Review, 114(2), 273-315.
Libben, G. (2006). Why study compound processing? In G. Libben & G. Jarema (Eds.), The representation and processing of compound words (pp. 1-23). Oxford: Oxford University Press.
Danks, D. (2003). Equilibria of the Rescorla-Wagner model. Journal of Mathematical Psychology, 47(2), 109–121.